The Epistemic Challenge Facing the Regulation of AI
Table of Contents
Josh Lee Kok Thong*Co-Founder and Editor of LawTech.Asia; Chairperson of the Steering Committee of the Asia-Pacific Legal Innovation and Technology Association; and Research Fellow of the Centre for AI and Data Governance in the Singapore Management University School of Law (SMU SOL). Graduated cum laude (with honours) from the SMU SOL in 2015, and holds an Advanced Diploma in Business Management from the Management Development Institute of Singapore. This piece is co-written in the author’s personal capacity. & Tristan Koh Ly Wey**Editor of LawTech.Asia; Undergraduate in the Double Degree Program in Law & Liberal Arts at Yale-NUS College and Faculty of Law, National University of Singapore. This piece is co-written in the author’s personal capacity.
The increased interest in artificial intelligence (‘AI’) regulation stems from increased awareness about its risks. This suggests the need for a regulatory structure to preserve safety and public trust in AI. A key challenge, however, is the epistemic challenge. This paper posits that to effectively regulate the development and use of AI (in particular, deep learning systems), policymakers need a deep understanding of the technical underpinnings of AI technologies and the ethical and legal issues arising from its adoption. Given that AI technologies will impact many sectors, the paper also explores the challenges of applying AI technologies in the legal industry as an example of industry-specific epistemic challenges. This paper also suggests possible solutions: the need for interdisciplinary knowledge, the introduction of baseline training in technology for legal practitioners and the creation of a corps of allied legal professionals specialising in the implementation of AI.
I. INTRODUCTION
The regulation of artificial intelligence (‘AI’) has been a hot topic in recent years. This may stem from increased societal awareness of: (a) the possibilities that AI may deliver across various domains; and (b) the risks that the implementation of AI may cause (e.g. the risk of bias, discrimination, and the loss of human autonomy). These risks, in particular, have led leading thinkers to claims that AI technologies are ‘vastly more risky than North Korea’1Samuel Gibbs, ‘Elon Musk: AI “Vastly More Risky Than North Korea”’ The Guardian (London, 14 August 2017) <www.theguardian.com/technology/2017/aug/14/elon-musk-ai-vastly-more-risky-north-korea> accessed 18 April 2020. and that there was a need to be ‘mindful of its very real dangers’.2João Medeiros, ‘Stephen Hawking: “I fear AI May Replace Humans Altogether”’ (WIRED, 28 November 2017) <www.wired.co.uk/article/stephen-hawking-interview-alien-life-climate-change-donald-trump> accessed 18 April 2020.
A key challenge facing policymakers creating regulations for AI (or, for that matter, any new technology), however, is the epistemic (i.e. knowledge-based) challenge. In short, policymakers must have domain knowledge in order to be able to sufficiently appreciate the scope, size, degree and impact of any regulation, and propose solutions that are effective and pragmatic. It has been recognised, however, that policymakers generally lack subject-matter expertise when crafting policies or regulations, especially in fields of scientific or technical knowledge.3Jeffrey H. Rohifs, Bandwagon Effects In High Technology Industries (MIT Press 2003) 49. To effectively regulate the development and use of AI, it is clear that policymakers and regulators will need to possess a deep understanding of AI technology and its technical underpinnings.4A Similar Call Has Been Made by the Gradient Institute’s Response to the Public Consultation for Australia’s AI Ethics Framework. See Tiberio Caetano and Bill Simpson-Young, ‘” Artificial Intelligence: Australia’s Ethics Framework” CSIRO’s Data61 discussion paper’ (Gradient Institute, 5 April 2019) <https://gradientinstitute.org/docs/GI-AustraliaEthicsAIFrameworkSubmission.pdf> accessed [please insert date here], where it is mentioned that ‘To drive and measure ethical intent, proper societal, institutional and legal accountability mechanisms need to be developed. It is crucial that such mechanisms, when developed, are properly technically informed by considerations of how AI systems actually operate.’ Similarly, for legal practitioners, having insufficient understanding of AI may result in challenges applying or advising on these technologies in practice.
Given the existence of various kinds of AI technologies, this paper focuses on neural networks, a particular type of machine learning technology used in deep-learning AI systems. In this regard, this paper highlights several key ethical and legal issues that could result from the adoption of AI, including the apportionment of legal liability, issues with accountability, bias, and ethical issues in legal practice. We also suggest possible solutions to address these issues: first, the need to grow interdisciplinary knowledge to build explainable AI; second, the introduction of baseline training in technology for legal practitioners; and third, the creation of a corps of allied legal professionals to serve as specialists in the implementation of technology in law to support the provision of legal services.
II. DEEP LEARNING AND NEURAL NETWORKS
Neural networks form the architecture of a particular recent type of AI technology known as ‘deep learning algorithms’. This form of AI technology has been represented in the media as being able to produce spectacular, almost magical, results in tasks previously thought unassailable by machines. AlphaGo Zero, famously known as the system that mastered the strategy game of Go, is a prominent instance of an AI system using deep learning that has received wide media coverage.5Will Knight, ‘AlphaGo Zero Shows Machine Can Become Superhuman Without Any Help’ (MIT Technology Review, 18 October 2017) <www.technologyreview.com/2017/10/18/148511/ alphago-zero-shows-machines-can-become-superhuman-without-any-help/> accessed 18 April 2020. See also Sarah Knapton, ‘AlphaGoZero: Google DeepMind Supercomputer Learns 3,000 Years of Human Knowledge in 40 Days’ The Telegraph (London, 18 October 2017) <www.telegraph.co.uk/science/2017/10/18/alphago-zero-google-deepmind-supercomputer-learns-3000-years/> accessed 18 April 2020. Deep learning systems have also made significant strides in natural language processing, a domain that is highly contextual given the subtleties of human language. For instance, OpenAI’s ‘GPT-2’ system is able to generate coherent bodies of text almost indistinguishable from natural human writing in response to short prompts. 6James Vincent, ‘OpenAI’s New Multimedia AI Writes, Translates, Slanders’ (The Verge, 14 February 2019) <www.theverge.com/2019/2/14/18224704/ai-machine-learning-language-models-read-write-openai-gpt2> accessed 27 April 2020. The system is also able to produce original fiction and non-fiction pieces of writing (e.g. short stories and newspaper articles). Given that the model was not trained specifically in any genre or particular type of writing, its flexibility in producing coherent text of varying tones and vocabulary demonstrates the versatility and potential of neural networks.7In fact, OpenAI withheld publicly releasing the algorithms behind the GPT-2 system for ten months from its announcement, for fear that the algorithms could be abused for non-benign purposes. See James Vincent, ‘OpenAI Has Published the Text-Generating AI it Said Was Too Dangerous to Share’ (The Verge, 7 November 2019) <www.theverge.com/2019/11/7/20953040/openai-text-generation-ai-gpt-2-full-model-release-1-5b-parameters> accessed 27 April 2020.
Deep learning algorithms are, generally speaking, ‘machine learning algorithms designed and structured in multiple levels or layers and are inter-connected, resembling a neural network architecture’.8Hannah Yeefen Lim, Autonomous Vehicles and the Law: Technology, Algorithms and Ethics (Edward Elgar Publishing Limited 2018) 89. This ‘neural network architecture’ comprises layers of interconnected nodes and branches (hence the name ‘neural network’, as the deep learning system mimics – superficially – the structure of biological brain neurons). Each layer of nodes processes a piece of information before the information is then passed to the next layer to process another aspect of it. The output of the system comes when the information has passed through all of the layers, with the results from the various layers adding up to a single output.9Luke Dormehl, ‘What is an Artificial Neural Network? Here’s Everything You Need to Know’ (Digital Trends, 6 January 2019) <www.digitaltrends.com/cool-tech/what-is-an-artificial-neural-network/> accessed 18 April 2020; see also Larry Hardesty, ‘Explained: Neural Networks’ (MIT News, 14 April 2017) <http://news.mit.edu/2017/explained-neural-networks-deep-learning-0414> accessed 18 April 2020.
An important distinction, however, should be drawn: deep learning algorithms are not the same as artificial general intelligence (‘AGI’). AGI is, put simply, machine intelligence that is able to perform any task that humans are generally able to do (e.g. playing a sport, reading a book, writing a story). This is a holy grail-esque vision that has yet to be attained and is widely believed to be decades, if ever, away from being realised. While current deep learning systems may be able to perform astonishing feats in specific limited domains (e.g. playing a game), these systems are not intelligent generally – they are unable to mimic, for instance, the ability of a three-year old to instinctively identify her mother or count the number of trees in a park.
III. THE LACK OF ‘EXPLAINABILITY’ OF NEURAL NETWORKS
To better appreciate the legal and ethical issues that could arise from the use of deep learning algorithms, a deeper exposition on the workings of neural networks is needed.
Like standard machine learning systems, neural networks also need to be trained before they can be effectively used. The difference, however, lies in how they are trained – while standard machine learning systems need to be trained with structured data to know categorisation features it should look out for, neural networks in deep learning systems are able to automatically discover such categorisation features, even if the data provided is unstructured. This is because neural networks are trained through the forward and backward propagation of values (i.e. information).
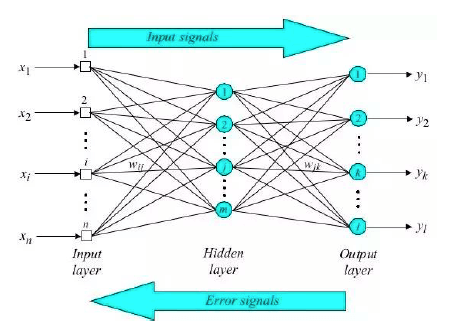
The propagation of values through a neural network system is better understood through a diagram, as seen above. Information enters the system (on the left of the diagram) and final estimates are returned (on the right of the diagram). To optimise the neural network, the final estimates from the right are propagated back towards the left. Depending on how close these estimates are to the actual values of test data, the individual weights of the nodes (i.e. the amount of emphasis placed on the output of each level of nodes) are tuned automatically by the network. In turn, such tuning improves the accuracy of the neural network’s results. Following repeated iterations, the network is ‘optimised’ by having the most optimal distribution of weights throughout the network that returns estimates closest to the actual values. It is this ability of neural networks to automatically tune their parameters that characterise neural networks as ‘self-learning’.
While literature on the optimisation of neural networks is extensive, conventional computing knowledge is still insufficient in explaining how neural networks are able to ‘self-learn’ and provide more accurate results than traditional machine learning techniques (to which end Max Tegmark, a physicist at MIT and author of Life 3.0, has posited that understanding this ‘self-learning’ ability requires not just knowledge of computing, but also physics).10Max Tegmark, Life 3.0: Being Human in the Age of Artificial Intelligence (Knopf 2017). This lack of explainability of neural networks has led to these networks being called ‘black boxes’, implying that it is difficult to predict results with confidence when parameters of the neural network are changed.
However, as will be shown below, the description above of how neural networks function reveals a significant consideration: as autonomously ‘self-learning’ neural networks may be, the ultimate trainer – a human – continues to play an indispensable role in ensuring that the neural network is trained to produce the most important results. This can be seen from how the neural network can only optimise itself based on test data. If the test data does not align with the desired output of the AI system, the neural network will produce an unwanted or erroneous outcome. For example, if AlphaGo Zero was trained on the basis that losing a Go game was a desired outcome, AlphaGo Zero would never be able to win any human player, regardless of how good (or bad) the player is or how many times the program tried. It is with this in mind that we turn to purported lack of explainability in neural network-powered systems and the legal and ethical risks posed.
IV. LEGAL AND ETHICAL ISSUES RAISED BY NEURAL NETWORKS AND DEEP LEARNING SYSTEMS
A. Legal issues
While the application of existing legal principles to neural networks would undoubtedly face a host of issues, one of the most evident would be the issue of remoteness of damage when finding liability under the tort of negligence. In this regard, the conventional test widely accepted in Commonwealth jurisdictions is whether the type of damage caused was reasonably foreseeable.11Overseas Tankship (UK) Ltd v Morts Dock and Engineering Co Ltd (The Wagon Mound No 1) [1961] UKPC 2, [1961] AC 388. It may be argued that this test is inappropriate when applied to neural networks. Specifically, given the supposed lack of explainability of neural networks, arguments could be made on the part of AI developers and deployers that the damage caused (if any) by the AI system would be too remote, given that they (i.e. AI developers and deployers) do not know how the neural network would optimise its internal values. For example, it may be argued that the type of damage was not reasonably foreseeable when a developer cannot ascertain with reasonable certainty how their self-driving car would behave when presented with unconventional scenarios (e.g. how the car would react when detecting defaced road signs).
Another issue called into question is the standard of care to be applied on AI developers or deployers in relation to neural networks. The question is whether the standard of care should be reduced, on the basis that the developer may not be able to foresee how its software would react in unconventional scenarios and that it would be unfair to impose a rigorous standard of care. Alternatively, the converse proposition could also be asked – i.e. whether the standard of care should be increased, precisely because more effort should be taken for AI developers to optimise their deep learning systems before releasing them into a live environment.
In the face of such issues, how should legal practitioners formulate their legal arguments for their clients in such novel situations? Some experts have argued that a possible solution to this quandary is to impose legal personality (whether full or partial) on the deep learning AI system, and to mandate that the AI systems are to be covered by insurance. This is so that the AI system may be sued, and the resulting damages, if any, can be paid by insurance companies. For instance, this has been proposed for AI systems in the MedTech, or medical technology, sector. 12Hannah R. Sullivan and Scott J. Schweikart, ‘Are Current Tort Liability Doctrines Adequate for Adressing Injury Caused by AI?’ (2019) 21(2) AMA Journal of Ethics <https://journalofethics.ama-assn.org/sites/journalofethics.ama-assn.org/files/2019-01/hlaw1-1902_1.pdf> accessed 14 April 2020. This solution, however, does not appear to be the most principled or intuitive on several levels. First, the proposal essentially shifts the practical burden of liability not to the AI system, but to insurance companies. Second, it is difficult to see how there is a need for the AI system to be imbued with some degree of personhood for incidents arising from their use to be covered by insurance – it would be sufficient to mandate that all incidents arising from AI systems would be covered by insurance without having to impose legal personality on AI systems. Indeed, this has already been enacted into legislation regarding autonomous vehicles in the UK under section 2(1) of the Automated and Electric Vehicles Act 2018, without recognising driverless systems as legal entities.13Automated and Electric Vehicles Act 2018 s 18. Third, based on first principles, it is difficult to see how imposing legal personality on AI systems would reduce their propensity to cause harm. These AI systems would not be able to automatically respond to the imposition of fault and liability the same way that human beings or corporations can – i.e. correcting their behaviour and making sure that the incident does not happen again. The idea of deterrence does not prima facie apply to AI systems that can function without human intervention. Fourth, this appears to merely kick the can down the road, as difficult questions relating to standards of care would continue to exist regardless of whether tortious liability was placed on human individuals and entities or AI systems.
Given that it is ultimately the humans training the deep-learning system that broadly have control over the output of the system, a justifiable and credible argument could, in our view, be made for the position that the party training the deep learning system (i.e. either the AI developer or deployer) should bear a certain amount of responsibility for the output of the system. It could be further argued that:
- A higher standard of care should be imposed upon this party to reasonably ensure that the output of the AI system is optimised, and that the AI system is not used in a live environment before it has been so optimised;14Hannah Yeefen Lim (n 8) 92. and
- That the damage suffered would have been reasonably foreseeable by the party responsible for training the system.
On the former point, given that the extent of optimisation of the deep learning system depends on how it is trained and the data it is given, and given that it is possible to visibly display every single processing step taken by a computer program (including those using machine learning algorithms),15ibid 89. it could be said that the degree to which a deep learning system is a black box is actually largely a function of the extent of the AI developer’s or deployer’s due diligence. The eventual standard of care could eventually be distilled down to a few elements, including doing risk impact analyses, conducting adequate testing and optimisation, and ensuring sufficiency and quality of the data used to train the deep learning algorithm. On the latter point, a policy argument could be made that the test for remoteness should be extended in the case of deep learning algorithms to acknowledge the proximity of the human trainer (be it the AI developer or deployer) to the damage. Given, as we have seen above, the criticality of the data input by the human trainer (and no other) to direct the optimisation of the AI system, it would be fair and justifiable to recognise that the trainer’s breach of duty was not too remote from the damage caused.
B. Ethical issues
Ethical conversations in relation to AI systems often fall into two categories. The first category comprises conversations that veer too much into the ethical realm (e.g. ‘should we let AI choose between killing five young children or one old grandmother?’ or the numerous other variations of the ‘trolley problem’). The second category comprise conversations that generally dismiss the ethical challenges posed by modern AI systems altogether as ‘soft’, baseless or premature. The discussion below aims to bridge both conversations by highlighting the importance of ethical discussions in relation to the development and use of AI systems, while being anchored in an epistemic appreciation of the technology. This is an important undertaking as ethics should be seen as ‘a guiding process in legal matters … (with) the ethical standard as a prefiguration of the legal standard’.16French Data Protection Society (CNIL), ‘How Can Humans Keep The Upper Hand? The Ethical Matters Raised by Algorithms and Artificial Intelligence’ (CNIL, December 2017) 24 <https://www.cnil.fr/sites/default/files/atoms/files/cnil_rapport_ai_gb_web.pdf> accessed 27 April 2020. This entails having an informed discussion in order to lay the foundational groundwork for the establishment of legal frameworks in the future. In this regard, several ethical issues will be discussed below: (a) bias; (b) accountability; and (c) ethical issues raised by the use of AI in legal practice.
1. Bias
A primary issue that deep learning systems powered by neural networks face is the issue of bias. At first blush, especially based on the example above, it appears that bias should not be an issue with neural networks. After all, as shown in the preceding section, instead of being trained to recognise patterns in gargantuan and biased datasets (as in the case of supervised machine learning), neural networks are trained by the deep learning algorithm making comparisons between its output and the training dataset and adjusting its weights accordingly. Indeed, in technical circles, bias is not recognised as a problem in neural networks, but as a necessary technical element in the form of the ‘bias node’ or the ‘bias neuron’. 17Oludare Isaac Abiodun, ‘State-of-the-Art in Artificial Neural Network Applications: A Survey’ (2018) 4(11) Heliyon <www.sciencedirect.com/science/article/pii/S2405844018332067> accessed 29 April 2020. In deterministic questions such as determining whether an image contains a cat, it is hard to imagine bias being an issue in deep learning systems – it is either right or wrong.
Experience, however, shows that deep learning systems too can entrench cognitive biases, to embarrassing effect. For instance, Google Photo’s deep neural network has been known for its success in image analytics. In June 2015, however, the same neural network gained notoriety when it labelled photographs of a black Haitian-American programmer in Brooklyn and his friend as ‘gorillas’.18Forrester Research, Inc., ‘The Ethics of AI: How to Avoid Harmful Bias and Discrimination’ (Forrester Research, Inc, 27 February 2018) <www.ibm.com/downloads/cas/6ZYRPXRJ> accessed 27 April 2020 In another example, a neural network predicting the income levels of individuals was shown to also be able to predict the race and gender of the same individuals, even though such attributes had been removed in the training dataset.19Jan Teichman, ‘Bias and Algorithmic Fairness’ (Towards Data Science, 4 October 2019) <https://towardsdatascience.com/bias-and-algorithmic-fairness-10f0805edc2b> accessed 14 April 2020. Such cognitive biases often arise when the neural network is trained on incomplete datasets, or because of ‘redundant encoding’ – i.e. training the neural network on variables acting as a proxy for another variable that should not be included (e.g. training the neural network on datasets that do not contain gender, but contain other attributes such as income levels that still reflect a gender bias). In the case of redundant encoding, the task of removing bias has been called ‘almost impossible’.20Forrester Research (n 19). Interestingly, in the case of the income prediction neural network, when another algorithm was used which had a reduced ability to identify race and gender, its accuracy of predicting income fell nearly by 50%.
Consequently, the main ethical question becomes whether deep learning systems that inadvertently exhibit bias should be trained and used in society, 21French Data Protection Society (n 17) 34. This is essentially the same question, posed differently: should we accept that AI only ever replicates bias and discrimination that are already ingrained in society? especially when decisions on how it is trained and used could entail making decisions about entrenching bias in an almost unconscious manner.22ibid 33. Answering this question will certainly not lend to black-and-white answers and in many cases, will be answered with a ‘it depends’. If the possibility is left open for deep learning systems that could exhibit and entrench bias to continue to operate, then based on the understanding of neural networks and deep learning systems above, regulators will need to deal with several other questions: (a) in what cases should such deep learning systems be considered acceptable for use in society; and (b) how can the cognitive bias of the system be minimised? Addressing these issues in real life could require mandating risk-impact assessments, the need to consider the extent of human oversight for such systems, and the putting in place of robust data management processes like ensuring data quality and understanding the lineage of data.
2. Accountability
Another primary issue is that of accountability. While definitions of accountability abound in different contexts, a general purpose definition in the context of AI can be taken as ensuring that ‘AI actors are responsible and accountable for the properfunctioning of AI systems and for the respect of AI ethics and principles, based on their roles, the context, and consistency with the state of the art’.23Info-communications Media Development Authority, ‘Model Artificial Intelligence Governance Framework Second Edition’ (Personal Data Protection Commission Singapore, 21 January 2020) <www.pdpc.gov.sg/-/media/files/pdpc/pdf-files/resourcefororganisation/ai/sgmodelaigovframework2.pdf> 64 accessed 14 April 2020. What follows from this definition of accountability are two considerations: how to implement accountability, and by how much.
The first question touches on the challenge of providing accountability (i.e. the ability to hold an actor responsible and accountable), even though deep learning systems are supposedly ‘black boxes’. Based on the preceding section, it appears that these ‘black boxes’ are not so after all, since it is possible to technically show precisely where and how a neural network in a deep learning system made a selection that led to an eventual decision. Nevertheless, technical possibility does not equate to practical feasibility. Examining line-by-line every output of the neural network (including after taking into account back-propagation) to identify the point where a particular turn was made could be beyond practicable human perception. Thus, while the deep learning system might not be a black box, it could be described as a ‘translucent box’ that remains practically inscrutable. In this regard, it has been remarked that transparency alone will not solve the issue of accountability – the AI system must be explainable in order for it to make sense not just to technical experts but also the lay person. Unless explainable, the question about whether accountability can be provided remains. Nevertheless, this appears to be the relatively more simple question to address. Explainability could be facilitated by proper communication based on context, proper documentation, and even the use of explainability tools (more on which will be discussed below).24Info-communications Media Development Authority (n 24) para 3.27.
The second question (or set of questions), on the other hand, is harder to answer. This is because it is context-dependent, and requires critical judgment calls. In particular, the ability of deep learning systems to mimic, replicate and/or replace the cognitive abilities of humans results in many areas which can be delegated to such systems, including form-recognition, decision-making and decision-support tools. With this, the following ethical questions arise, which must be addressed in order to answer two practical questions – whether to delegate decisions to deep learning systems, and if so, what is the degree of human oversight:
- A loss of accountability from traditional sources of accountability.25French Data Protection Society (n 17). Research has shown that the potential of deep-learning automated decision-making systems to perform better than human experts (e.g. in medical diagnosis), or to purportedly make decisions more efficiently and with less bias (e.g. in predictive policing, recidivism analyses in criminal justice systems, or decision-making of public institutions), tends to create over-reliance by humans on such decision-making systems. This could undermine traditional figures and institutions of authority, and undermine existing human-based problem-solving processes that we are accustomed to. This is especially when these figures and institutions of authority adopt deep learning systems without properly understanding how they function and should be integrated into existing decision-making processes. A separate but related concern is that such over-reliance could also result in an erosion of human expertise in the long run.26An example often raised is the tragically fatal Air France flight which crashed in 2004, where the pilots were unable to understand the flight status of the plane, when malfunctioning airspeed indicators caused the plane’s autopilot systems to stop functioning.
- A transfer of responsibility to unaccountable actors.27French Data Protection Society (n 17). The fact that deep learning systems introduced into live environments are trained by the AI developer or deployer means that ethical dilemmas and choices – such as the ‘trolley problem’ of whether a driverless vehicle has to kill its occupant or a pedestrian on the road28The Massachusetts Institute of Technology has designed a website that tests people’s moral intuition in variations of such a scenario, focusing on nine factors that include: sparing humans (versus pets), sparing passengers (versus pedestrians), sparing pedestrians who cross legally (versus jaywalking). The research collected nearly 40 million decisions from 233 countries. The three strongest preferences were sparing humans over animals, sparing more lives, and sparing young lives. While the first two are less contentious, the researchers note that the third preference conflicted with Ethical Rule 9 proposed in 2017 by the German Ethics Commission on Automated and Connected Driving. The rule states that any distinction based on personal features, including age, should be prohibited. Consequently, if such a preference were to be pre-programmed (or not), policymakers may face a backlash from the populace because of the conflict with public’s moral intuitions. – are moved from a person directly involved to a choice made by other actors elsewhere and well beforehand. To add to this concern, choices could be made without a proper understanding of the situation on the ground. Worse still, these choices could incorporate bias (whether social, political, ethical or moral) and are intended towards extrinsic purposes such as commercial choices for the deep learning system’s designers. In short, deep learning developers and/or deployers may not be suitably positioned or equipped to make such ethical decisions with, and could end up making decisions that contradict public policy or sentiment.
- The balance between accountability and utility.29French Data Protection Society (n 17). This issue touches on how much emphasis society should place on accountability, versus other priorities such as utility. Specifically, if society is concerned about accountability gaps, should society only use deep learning systems when we can ensure that liability and/or responsibility can be attributed with absolute certainty? While doing so would ensure no accountability gaps, this would be a long-drawn process (with potentially no end). This could in turn deny society the benefit of deep learning systems, given that we know that these systems can already outperform humans in most areas and do so more efficiently.
3. Legal and ethical issues in legal practice
The fact that AI systems can be used in numerous aspects of legal services has been one of the key drivers of the legal technology industry (i.e. the industry where existing and emerging technologies are developed to improve and transform the delivery of legal services). The ways in which AI systems are being used in legal service delivery today include: technology-assisted review (i.e. the use of technology solutions to organise, analyse and search very large and diverse datasets for e-discovery or record-intensive investigations); legal analytics (i.e. the use of big data and AI to make predictions from or detect trends in large datasets, such as for practice management and even litigation outcome management); practice management assistants (i.e. the use of AI’s natural language processing abilities to manage, review and research information); and legal decision-making (i.e. the use of AI to assist in judicial or administrative decision-making). The transformative potential of AI systems in the legal industry has in fact led to a commentator to say that ‘(a)rtificial intelligence is changing the way lawyers think, the way they do business and the way they interact with clients. Artificial intelligence is more than legal technology. It is the next great hope that will revolutionize the legal profession.’30Julie Sobowale, ‘Beyond Imagination: How Artificial Intelligence Is Transforming the Legal Profession’ [2016] ABA j. 46, 48.
Described in such optimistic terms, it is advised that legal professionals begin to turn their minds to the possibility that AI systems, especially deep learning systems, will be ubiquitously utilised in many areas of legal service delivery in the future. While some of the ways in which AI systems are presently used in legal service delivery utilise machine learning systems (as opposed to deep learning systems), it is not unthinkable that deep learning systems can eventually be trained to match or even outmatch the performance of machine learning systems (if AlphaGo Zero is anything to go by). If so, the legal and ethical issues raised in the preceding paragraphs will arise as issues that will need to be addressed by the legal profession. For instance:31Gary E. Marchant, ‘Artificial Intelligence and the Future of Legal Practice’ (The SciTech Lawyer, 2017) <www.americanbar.org/content/dam/aba/administrative/litigation/materials/2017-2018/2018sac/written-materials/artificial-intelligence-and-the-future.authcheckdam.pdf> accessed 30 April 2020.
- Will the use of deep learning AI systems open lawyers and law practices to charges of the unauthorised practice of law? For instance, an online system known as ‘Ross’, which has been called ‘the world’s first robot lawyer’, is an online research tool using natural language processing technology to research and analyse cases and is presently in use in several US law firms. Depending on the level of human oversight over the output of Ross, could law firms be accused of facilitating the unauthorised practice of law?
- Given the self-learning capability of deep learning systems, how can lawyers ensure the accuracy, legality and fairness of the AI systems output, considering the professional standards that lawyers are legislatively held to? Further, could lawyers be held liable in negligence when mistakes are made?
- Will lawyers be liable for malpractice for not using AI that exceeds human capabilities in certain tasks? This is a question that is faced not just in legal practice, but in other professions such as medicine. The issue at hand is whether it would be unethical not to use a deep learning AI system, when the system has consistently shown to outperform human lawyers at a particular task. For instance, in a widely-publicised event, an AI system from LawGeex, a legal technology company, outperformed 20 experienced lawyers in reviewing risks in non-disclosure agreements. The AI system had an accuracy level of 94%, while the lawyers had an average accuracy level of 85%. What was more significant was that the AI system performed the task in 26 seconds, as compared to the average speed of 92 minutes for the lawyers.32Johnny Wood, ‘This AI Outperformed 20 Corporate Lawyers at Legal Work’ (World Economic Forum, 15 November 2018) <www.weforum.org/agenda/2018/11/this-ai-outperformed-20-corporate-lawyers-at-legal-work/> accessed 30 April 2020.
- If deep learning systems begin to replace more routine tasks in legal practice traditionally performed by young lawyers, how will this affect their training? Could this lead to an over-reliance on such systems and a reduction in the standards of the profession over the long run?
V. BUILDING SOLUTIONS TO THE PROBLEM
A. Explainable AI
In response to the epistemic challenges to regulating deep learning AI systems, there is a growing awareness about the need for ‘explainable AI’, a concept that has developed in response to the relative lack of understanding of deep learning systems. At its heart, explainable AI is a principle to ensure that decisions made by AI systems and the associated data driving those decisions can be explained to end-users and other stakeholders.33Info-communications Media Development Authority (n 24) 20. This translates into design principles, techniques and tools that provide insights into how neural networks make decisions.
With regard to principles and techniques, the Model AI Governance Framework issued by Singapore’s Personal Data Protection Commission, for instance, provides guidance on steps that organisations can take to improve their ability to explain how an AI system arrives at a decision. One step suggested is to ‘incorporate descriptions of the solutions’ design and expected behaviour into their product or service description and system technical specifications documentation to demonstrate accountability to individuals and/or regulators’.34Info-communications Media Development Authority (n 24) 13.
With regard to tools, Google’s TensorFlow Playground allow researchers to adjust parameters in a neural network, providing a simple and graphical way to explain how a neural network arrives at a decision.35TensorFlow, ‘Tinker with a Neural Network Right Here in Your Browser’ (TensorFlow) <https://playground.tensorflow.org/#activation=tanh&batchSize=10&dataset=circle®Dataset=reg-plane&learningRate=0.03®ularizationRate=0&noise=0&networkShape=4,2&seed=0.14888&showTestData=false&discretize=false&percTrainData=50&x=true&y=true&xTimesY=false&xSquared=false&ySquared=false&cosX=false&sinX=false&cosY=false&sinY=false&collectStats=false&problem=classification&initZero=false&hideText=false> accessed 14 April 2020. IBM’s AI Fairness 360 Open Source Toolkit also provides open source code for developers to detect and correct possible biases in predictive analytics models.36IBM, ‘Trusting AI’ (IBM) <www.research.ibm.com/artificial-intelligence/trusted-ai/> accessed 14 April 2020. Nevertheless, it may not always be possible to fully rely on technological tools to identify ethical shortcomings in deep learning systems. IBM notes that while its toolkit covers over 70 metrics of ‘fairness’, the toolkit is still unable to identify all possible aspects of fairness in all situations. Further, the use of one metric over another in the toolkit is highly context-dependent and still requires human judgment.37IBM, ‘AI Fairness 360 – Resources’ (IBM) <http://aif360.mybluemix.net/resources#guidance> accessed 14 April 2020. In this regard, as with many cases, these solutions are but tools to aid, but not entirely replace, the human capacity to act ethically.
Further, in our view, explainable AI forms just one portion of a dual-faceted approach to breaking down the regulation of AI:
- Ascertaining how the AI system functions. This relates to the epistemic issues in relation to AI systems, some of which have been identified above. Explainable AI is part of the larger solution to understanding these epistemic issues, and will require an understanding of computer science, physics, mathematics and other domains.
- Ascertaining a desirable outcome for AI systems. Other than having explainable AI, fuller consideration needs to be given to ethical dilemmas posed by the use of deep learning systems. For instance, should we maximise the survivability of passengers or pedestrians in a driverless car? Should we allow governments to implement facial recognition software that is justified by prioritising the safety of its citizens? These are normative questions best answered by legal practitioners, government policy makers and moral philosophers in conjunction with technical expertise from AI engineers. After all, we should not be holding AI systems to the heights of ethical standards, when we humans have yet to fully debate and find solutions to these issues.
In relation to the point on ethically desirable outcomes, it is heartening to note that the present lacuna in multi-disciplinary research in AI governance is rapidly being filled. One group involved in such research is The Future of Humanity Institute at Oxford University which ‘strives to help humanity capture the benefits and mitigate the risks of artificial intelligence’.38Future of Humanity Institute, ‘Centre for the Governance of AI’, (University of Oxford) <www.fhi.ox.ac.uk/govai/#home> accessed 14 April 2020. Its researchers range from various disciplines, such as philosophy, economics, political science, computer science and law, recognising that AI governance is not the province of only one discipline. Singapore itself is an emerging thought leader in this space, with the Centre for AI & Data Governance at the Singapore Management University39Centre for AI and Data Governance, ‘About’ (Singapore Management University) <https://caidg.smu.edu.sg/about> accessed 14 April 2020. and the recent establishment of the Centre for Technology, Robotics, Artificial Intelligence & the Law at the National University of Singapore.40Centre for Technology, Robotics, AI and the Law, ‘About TRAIL’ (National University of Singapore) <http://law.nus.edu.sg/trail/> accessed 14 April 2020. Addressing such ethical dilemmas will allow us to better deal with the typical ‘trolley problems’. If a scenario can be predicted, its occurrence assessed to be sufficiently likely and the risks associated with its occurrence sufficiently non-trivial, we can design appropriate safeguards or responses to ensure that our AI systems deal with these situations. While it is not possible to cater for all scenarios, some of the more likely or higher-risk scenarios would be foreseeable, and can be addressed by designing AI systems to design specific responses.41Yeong Zee Kin, ‘Legal Issues in AI Deployment’ (Law Gazette, February 2019) <https://lawgazette.com.sg/feature/legal-issues-in-ai-deployment/> accessed 14 April 2020.
B. Expansion of knowledge in the intersection of law and technology
The epistemic challenge can also be addressed by raising the awareness of fundamental computing and technological concepts within the legal profession. Providing such education will allow legal professionals a baseline capability to engage in and address legal and ethical issues, and is a powerful tool for policymakers. In this regard, two suggestions are proposed: (a) introducing mandatory baseline training in AI for legal professionals; and (b) the creation of a corps of allied legal professionals (‘ALPs’) that have professional expertise in both technology and law to create a sustainable talent pool.
C. Baseline training in AI for all legal professionals
Given the wide scope of ethical and legal issues that AI potentially brings to the legal industry and beyond, it is proposed that baseline training in legal issues around technology be mandated as part of the requirements for becoming professionally qualified to practise law. This would raise the technical awareness of technology within the profession, as well as the legal issues that could arise. Doing so would be a timely and foresighted step, given the range and extent to which technology has pervaded almost all areas of industry and practice areas. The content of such training could be similar to the online course CS50 Introduction to Computer Science for Lawyers run by the Harvard University, which covers fundamental concepts in computing (e.g. algorithms, cloud computing, databases, privacy, security) taught from the context of the legal profession to enable lawyers to understand the work of developers and how technological solutions deployed could impact clients.42Doug Lloyd and David J. Malan, ‘CS50’s Computer Science for Lawyers’ (Harvard University) <https://cs50.harvard.edu/law/> accessed 14 April 2020. Avenues that such a course could be incorporated into include the Bar Examinations curriculum that law graduates must pass to be called to the Singapore Bar.
D. Creating a corps of allied legal professionals
The creation and recognition of a professional corps of ALPs could be another important component of the infrastructure needed to tackle the epistemic challenge of AI and deep learning systems. In brief, ALPs are professionals that act as support for the practicing lawyer such that the lawyer can focus on the provision of legal advice.43Future Law Innovation Programme (FLIP), ‘Legal Engineer: Process Automation Wizards’ (Singapore Academy of Law, 10 February 2020) <www.flip.org.sg/post/legal-engineer-process-automation-wizards> accessed 14 April 2020. While the definition of FLIP covers individuals who focus primarily on business process automation, there is no reason why it cannot or should not extend to providing expertise in the realm of ethics. This support can take the form of technical, business or even ethical expertise. Having such a corps in professions is not a new concept – in healthcare, the importance of allied health professionals working alongside professional doctors in Singapore has been legislatively recognised since 2013.44See Allied Health Professions Act (Cap 6B, 2013 Rev Ed). The idea of having a corps of ALPs has also been judicially suggested as recently as 2019.45Chief Justice Sundaresh Menon, ‘Deep Thinking: The Future of The Legal Profession in an Age of Technology’ (29th Inter-Pacific Bar Association Annual Meeting and Conference, Singapore, 25 April 2019) para 15 <www.supremecourt.gov.sg/docs/default-source/default-document-library/deep-thinking—the-future-of-the-legal-profession-in-an-age-of-technology-(250419—final).pdf> accessed 2 May 2020. Just like how a diagnostic radiographer is a specialist in using radiation equipment to aid the diagnosis, ALPs would act as specialists in their relevant fields to aid the provision of legal advice.
In the field of ALPs, there can be professionals whose role (presently performed by a group of people known as ‘legal technologists’ and ‘legal engineers’) that focus on the implementation and implications of technology and AI in the legal sector. These professionals would be part of the law firms and act as the central node for any issue relating to technology. Given their possession of technical expertise, these individuals would also be well-placed to understand the context and contribute to the discussion of ethical issues relating to technology and the practice of law. In short, such ALPs would act specialised ethicists in the legal industry, assisting lawyers to get a fuller technical and ethical grasp on issues to which legal concepts can be applied. This expertise could also be applied in-house, in relation to the law firm’s technology and innovation projects. For instance, for a law firm using a deep learning technology system for litigation outcome prediction, an ALP could help lawyers in assessing and minimising bias in data used to the system, minimising the risk that the output of such systems would be biased or discriminatory.
The professionalisation of this corps of ALPs is a potential policy solution which would raise the significance, profile and recognition of the contributions of this group to the legal profession, and in turn add as an incentive for the technical and ethical standards and contributions of these professionals to be raised as well.
VI. CONCLUSION
In summary, there needs to be a more holistic approach to the regulation of AI, particularly with deep learning systems and neural networks. The technology itself, while inaccurately described as a ‘black box’, still functions in ways that are practically unexplainable (made worse by the fact that legal professionals seldom have the technical knowledge to understand the technical underpinnings of such systems). This paper, with its call to improve the epistemic understanding of deep learning systems amongst relevant stakeholders, has endeavoured to shed some light on the technical aspects of deep learning systems and the neural networks that powers them, and has highlighted some of the key legal and ethical challenges presented. The final section of this paper proposed means by which these epistemic issues could be solved, at two levels: society and the legal industry. First, the epistemic issues can be addressed by increasing research into explainable AI as well as having programmes that study the complex legal and ethical issues brought about by the use of deep learning systems. Second, the raising of baseline technological awareness amongst lawyers and the recognition of a specialised group of ALPs which can provide lawyers with better assistance in dealing with technical and ethical issues. It is the authors’ hope that this paper will raise awareness about the epistemic challenge concerning deep learning AI systems, and spur the necessary ethical and policy debates required to solve some of the deeper questions highlighted above.
- 1Aqua Coder, ‘What is an Artificial Neural Network?’ (Aqua Coder) accessed 14 April 2020.